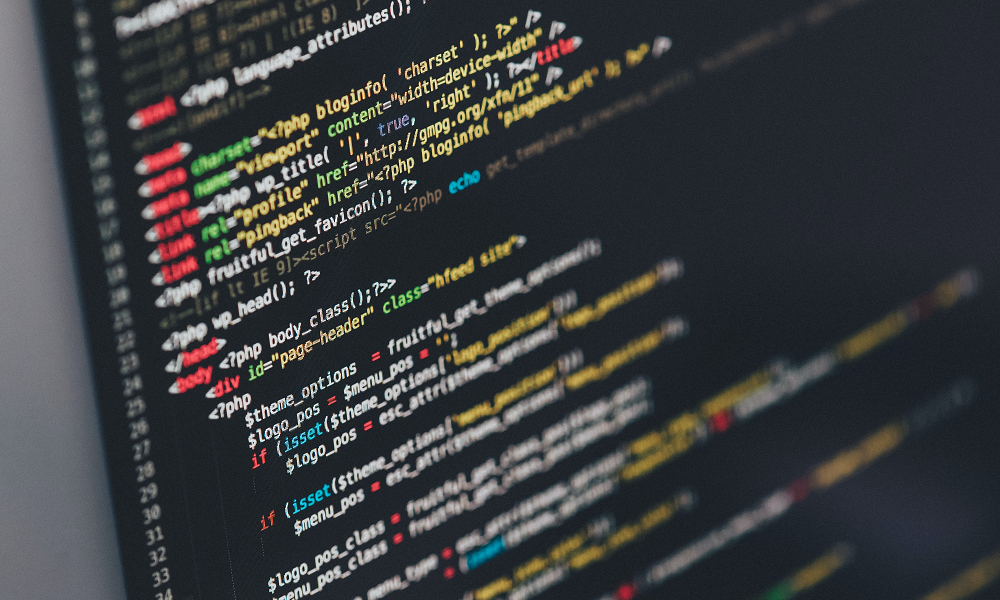
Formalin fixation of paraffin-embedded tissue samples is widely used to preserve tissue for clinical investigation. However, the integrity of the genomic material extracted from such samples is not always preserved, and it can lead to errors in downstream sequencing analysis, including somatic variant calling. GSC scientists conducted a comprehensive comparison of variant caller performance and established a bioinformatics-based approach to improve somatic variant calling from FFPE tissue samples.
Formalin-fixed, paraffin-embedded (FFPE) versus fresh frozen (FF) tissue samples
Tissue samples and their nucleic acids can be preserved through various means. Formalin fixation of paraffin-embedded tissue is a routine preservation method established for clinical purposes, such as pathology assays. Alternatively, flash freezing can preserve fresh tissue to yield high-quality nucleic acids. Many next-generation sequencing (NGS) studies, which require well-preserved genomic information, use whole blood or FF tissue samples.
Due to several logistic issues, such as procuring fresh tissue and having the specific infrastructure for storage, FFPE tissue samples are the preferred method for preserving clinical samples. At the same time, FFPE tissue is not the best starting material for NGS, as formalin fixation can result in nucleic acid damage. This damage, or lack of efficient preservation, can produce downstream genome analysis artifacts and confound the identification of somatic mutations/variants.
Genome sequence analysis: somatic variant calling
In the context of cancer, somatic mutations are genetic variants uniquely present in a tumour and not present in a patient’s constitutional DNA. Detecting somatic variants arising in DNA sequencing reads has become easier thanks to the advent of NGS technology.
Somatic variant calling is the approach used to detect or “call” the true, biological somatic mutations observed in sequencing data. Several types of mutations can be identified, including, but not limited to, structural variants (SVs), insertions and deletions (indels), and single nucleotide variants (SNVs). Sometimes, due to how sequencing data is acquired/assembled using NGS or the quality of the genomic starting material, sequencing artifacts may arise and be mistaken for true somatic mutations/variants.
To distinguish the true, biological somatic variants from the artifacts, multiple bioinformatics tools (referred to as “variant callers”) have been developed. In addition, approaches to filter or reduce somatic variant artifacts while retaining true sequence variants are also available.
Using machine learning to improve somatic variant calling from FFPE samples
To improve somatic variant calling from FFPE samples, several researchers, including lead author Dollina (Dolly) Dodani (a former GSC co-op student and current graduate student in the Talhouk lab at OVCARE), Matthew Nguygen (bioinformatics graduate student at UBC), GSC scientists Drs. Ryan Morin and Marco Marra, and process development coordinator Richard Corbett used whole-genome sequencing data extracted from FFPE and matched FF tissues to optimize an SNV calling approach for FFPE samples. This approach, along with a comprehensive comparison of different variant callers, was published in Frontiers in Genetics.
By comparing the data between FF and FFPE samples, the researchers were able to identify false-positive SNVs that arise uniquely in the FFPE samples and develop a computational solution to reduce their prevalence. They created FFPolish, a machine learning-based computational tool that can filter out FFPE-specific false positive somatic SNV calls.
FFPolish involves using input (FFPE and FF variants called by various callers) to generate or build a trainable machine learning model, which can then be fed new FFPE data to produce the output—a filtered list of called FFPE variants.
The researchers also provided a comprehensive comparison of different available variant callers to help the user identify which tool may be preferred for calling somatic SNVs from FFPE samples in specific applications. In the same work, they also offer the tool FFPolish to improve somatic variant calling from genomic data extracted from FFPE tissue samples. Corresponding author Richard Corbett believes that “as Fresh Frozen tissues become increasingly pauce, improvements to FFPE analysis methods are paramount”.
Learn more:
Learn more about machine learning in a broad sense here.
Learn more about the ongoing research by the Marra Lab at the GSC.
Learn more about the ongoing research by the Ryan Morin Lab at Simon Fraser University.
Acknowledgements:
This study was supported financially by funds from the National Institutes of Health (NIH) National Cancer Institute.
Citation:
Dodani DD, Nguyen MH, Morin RD, Marra MA, Corbett RD. Combinatorial and machine learning approaches for improved somatic variant calling from formalin-fixed paraffin-embedded genome sequence data. Frontiers in Genetics.
*bold font indicates members of the GSC.